The NHS could be well-placed to realise the benefits of AI in healthcare, writes Google Health’s Michael Macdonnell.
Imagine a world in which a simple scan could predict that one of your parents was losing their vision in time to prevent it from happening. Imagine if doctors could predict that your kidneys were at risk of damage severe enough to require dialysis in time to take corrective action. Imagine if your partner’s care team could foresee changes in the potassium in his blood before they become dangerous. Now imagine if these advances could be offered by the NHS.
They could be. Together with Moorfields Eye Hospital, we have developed an early stage AI-powered system that interprets Optical Coherence Tomography retinal images as well or better than specialists who have worked in the field for many years. This system triages fifty common diagnoses, correctly identifying the signs of sight-threatening disease. Taken by many high street opticians, OCT images are often referred to specialists – Moorfields alone receives more than 1,000 a day. This leads to unnecessary delays.
Just a few weeks ago, we published research conducted at the US Department of Veterans Affairs (the VA) in Nature. This AI system accurately predicted acute kidney injury – an emergency that kills 100,000 people in the UK alone – up to 48 hours before it shows up blood results. The model correctly predicted 90 per cent of patients whose condition deteriorated so severely that they later required dialysis. And, in a lesser noticed aspect of the research, the AI could also predict future blood potassium and urea levels.
Today, DeepMind Health is formally joining Google Health to help make this promise a reality. By drawing on Google’s global expertise and resources, we can more quickly translate groundbreaking research into tools and technologies that can save lives.
The NHS is well-placed to realise the benefits of AI in healthcare. Google Health is committed to translating our promising research into clinical tools that improve or even save lives
All right, lay off the techno Kool Aid I hear you say. You’re right to be a little wary. These studies were retrospective; that is, the algorithms were trained and then (separately) tested on historical data. Although subjected to peer review in premier scientific journals, they still require prospective validation. In so doing, we need particularly to be alive to the risks of bias. For example, the VA dataset is mainly male, reflective of the VA population. As with all deep learning models, more representative data is required before extending the model to other populations.
Besides, some proponents of AI sound better trained in hyperbole than in data science. AI will not replace doctors and nurses, as some wilder voices proclaim. These technologies will, however, augment clinicians’ skills, helping them to make better decisions rooted in a wider field of data than any single mind can process. In this respect, the impact of AI in healthcare could be similar to the advent of empirically-grounded treatment protocols or of modern imaging techniques.
But this time it’s personal. The AI systems we’re developing do not simply categorise patients or stratify their risk. The VA study makes inferences about specific cases based on data from 704,000 patients with over six billion clinical-event entries - from vital signs to lab results, from medication histories to clinical notes. They are predictions about individual people that enable personalised actions.
Translating insight into action is the hard part. Clinicians are overworked and overwhelmed. Technology can sometimes make things worse. This is why we’ve built Streams – a mobile assistant for clinicians. Streams serves clinical intelligence to doctors and nurses when they need it, whether at the bedside, on their rounds or on the run.
It’s showing promising results. Last month, a peer-reviewed evaluation demonstrated that kidney specialists using Streams at the Royal Free London reviewed urgent cases of AKI in minutes, rather than hours, and missed fewer cases overall (3.3 per cent rather than 12.4 per cent). Streams also reduced the average cost of admission for a patient with AKI by 17 per cent. This suggests implementation is cost-effective, considering that AKI costs the NHS more than £1bn billion each year.
Streams doesn’t yet anticipate AKI, although it does help clinicians react faster to its warning signs. In order to replicate the predictive power implied by our work with the VA, learning algorithms need to be applied to a much wider range of data that flow freely between care settings. Though similar to the VA in many ways, joined up data is still a long way off in many parts of the NHS, constituting a major barrier to cutting-edge AI technologies. Sustained, smart investment is needed in the nation’s health IT infrastructure.
Companies working in this space have not always got everything right and we’ve certainly learnt from our experience. We are committed to the highest standards of data security and privacy. We work closely with the clinicians and patients our technology is built to serve. We have also learned the importance of transparency.
The NHS is well-placed to realise the benefits of AI in healthcare. Google Health is committed to translating our promising research into clinical tools that improve or even save lives. In partnership with the NHS, I believe we can hasten an era of predictive medicine.
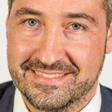
No comments yet