In these extracts from his series of articles for HSJ, Jim Lane gives insights on essential aspects of data modelling, including scoping the model, defining the baseline and visualising data
We’re living in an era where data has become a crucial element in the decision-making process. It’s particularly important in healthcare settings to help determine the best way to allocate resources in light of multiple converging trends, which present enormous challenges to healthcare leaders. The cost of care is rising along with a simultaneous increased demand for services by an ageing population – at the same time the available workforce is diminishing.
I’ve worked closely with NHS clients for years, producing data models and analytics. Lessons from my experiences help shed light on some essential aspects of data modelling, including scoping the model, defining the baseline and visualising data. These insights can help ensure that you get the information you need to make informed decisions that will impact the future of healthcare.
Scoping the Model
The key to successful data modelling begins with asking the right questions. Many tenders state the need to understand how many inpatient beds will be required in the future. Yet it is essential to look deeper – why do you need to know this? Are you building a business case, informing a one-off report or supporting operational performance?
Probing further provides context and determines additional criteria that will impact the model design, such as:
- How far in the future do you need to model?
- Should you differentiate at specialty/diagnosis level or different types of patients?
- Is patient age and gender important?
- Do you need to consider moving activity from one hospital to another or repatriating activity from other providers?
- Do you need to address average length of stay, or changes to the distribution of length of stay?
- Are there dependencies such as theatres and diagnostics to consider?
- Do you need to understand outputs by day/week/month/year?
It’s also important to understand who will use the model, their skill set, how they will access information and the technology systems they use. Public sector organisations may not have the advanced computers required to run complex Excel models, therefore impacting the way the model is developed.
By completing a comprehensive scoping exercise, you will have a framework to define the scale of the project and ensure the model fits the intended purpose. Specificity has another advantage: it can save money by narrowing the scope of work. There’s no need to build a palace when all you need is a shed.
Determining Baseline Data
A model without an agreed-upon baseline is akin to building a house on sand; it will eventually crumble and fall. Experience demonstrates that it’s challenging for stakeholders in healthcare environments to agree on a baseline position because people look at data differently, depending on their role. Clinicians tend to focus on patient procedures and diagnoses; financial managers concentrate on activity that generates revenue or incurs cost; performance and general managers may want to examine a patient’s entire stay (eg a spell). All of these factors could be relevant, depending on the situation and the questions you want to answer.
To achieve success, constituents must agree on the rules related to data processing, as well as caveats. Among the guidelines to consider:
- Be explicit about what are you counting and related currencies. For example are you using spells or episodes, attendances or appointments, procedures or sessions?
- Use common language, clarify the rules and review references applied to raw data. For instance, how are “patient types” defined? While day case and elective patients might be obvious, how are non-elective patients addressed?
- Ensure clarity on the time period the baseline covers and how activity is counted. Is it based on admissions or discharges? Are there open activities, which may not be included?
- Ensure the baseline period is current. The more time elapsed between the baseline end date and the processing date, the greater the risk of divergent opinions.
- Consider whether to make adjustments to the assumptions to reflect “normal” activity levels in subsequent years. My recommendation is that the baseline should not be adjusted – it should always reflect what actually happened.
From a modelling perspective, it’s important to determine the level of aggregation required to make sense of the data. Greater aggregation enhances efficiency, which may be relevant for more complex models. Because stakeholders typically assess the baseline at a level of aggregation that makes sense to them, consider cascaded outputs, which reflect different views on the data to build a story.
One side note regarding data collection. It’s vital to ensure that the requested data is provided in sufficient detail to allow you to model the scoped assumptions. For example, if one of the assumptions pertains to gender, this detail must be addressed.
However, data governance is taking on greater prominence, particularly in the healthcare sector due to the sensitive nature of this information. You should, therefore, ensure that any data request is proportional to the modelling requirements. If in doubt, check with sources such as the Office of the Information Commissioner (UK specific), particularly in the context of the General Data Protection Regulation, which begins enforcement in May 2018.
New Vistas in Visualisation
They say that a picture paints a thousand words. By the same token, a well-constructed visualisation can paint a thousand rows of data. There are now many options for presenting data in an appealing manner.
While it is unlikely you’ll design this yourself, you should understand the needs of the end-user, and communicate this to the person responsible for designing the display. A good visualisation maximises the reader’s cognitive ease, clearly and concisely articulating the message. The best presentations tell a story encompassing the following criteria:
- Insightful, action-oriented, support problem solving.
- Intuitive and self explanatory.
- Technically elegant and eye-catching with visual hooks to draw people in.
- Clearly marked, legible and annotated if possible.
- Provide information that isn’t obvious from simply examining the underlying data.
- Clearly indicate what is good/bad at a glance.
- Select the right type of graphics for your audience. Sometimes, a simple bar chart is all that’s required.
Beyond these details, consider how the data will be communicated. Will someone present this information and answer questions, or will it be accessed online or in a report? Put yourself in the shoes of the target audience, think about the questions they might ask, then build the answers into the narrative. Consider testing the visualisation before determining the final form.
An individual visualisation doesn’t need to answer every question in detail, but it should shine a light on where you may need to look. Above all, circle back to your starting point in the data modelling process. Revisit the questions asked when the model was scoped. If the visualisation answers or supports the questions at hand, you’ve done your job.
To read Jim Lane’s articles in full click here
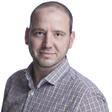
No comments yet